醫學徵象
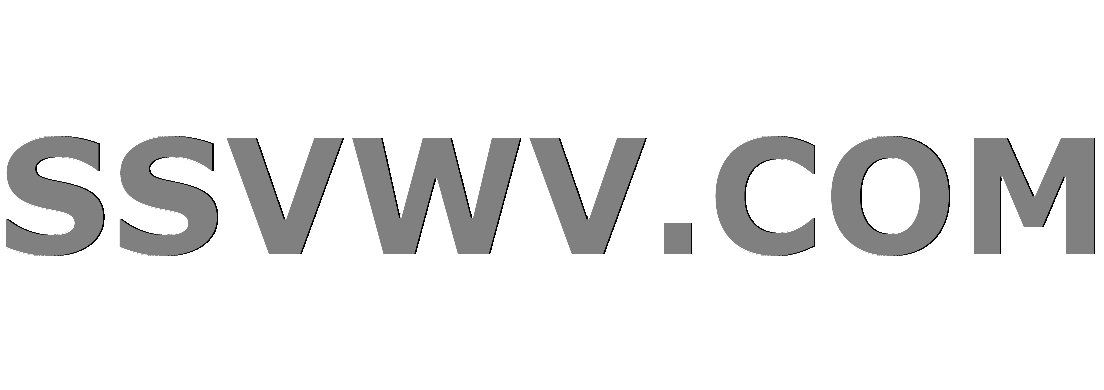
Multi tool use醫學徵象(英语:Medical sign),又稱體徵、病徵[1],醫學術語,指在進行身體檢查或病理檢查時,能夠提供醫生對醫療進展及疾病狀況的跡象及指標,通常是可客觀測度得到的。
「體徵」與「症狀」或「徵狀」不同,「症狀」或「徵狀」來自於病患主觀的感受,例如麻痹;但「體徵」則是可客觀觀察到的,例如肌肉紅腫。有些體徵對病人來說可能是無意義,甚至自己察覺不到的,例如高血壓;但是對於醫生診斷病人病情發展,及提供醫療照顧方面,是很重要的參考指標。
另見
註釋
^ http://shatinsj.org/firstaid/fa006.php
病理学:醫療條件和ICD碼
|
---|
| (疾病 / 失調 / 症候群 / 序列、症狀 / 醫學徵象、創傷等) | | (A/B, 001–139) | 傳染病/感染:細菌性疾病
- 病毒性疾病
寄生虫病
- 真菌病
- 人畜共通傳染病
|
---|
| (C/D, 140–239 & 279–289) |
癌症(C00–D48, 140–239) | |
---|
| 髓血液病(D50–D77, 280–289) | |
---|
| 淋巴免疫 (D80–D89, 279) | |
---|
|
---|
| (E, 240–278) | |
---|
| (F, 290–319) | |
---|
| (G, 320–359) | |
---|
| (H, 360–389) | |
---|
| (I, 390–459) | |
---|
| (J, 460–519) | |
---|
| (K, 520–579) | Oral and maxillofacial pathology
- Tooth disease
- salivary gland disease
- tongue disease
Digestive disease
- Esophageal
- Stomach
- Enteropathy
- Liver
- Pancreatic
|
---|
| (L, 680–709) | - 皮膚狀況
skin appendages
- Nail disease
- Hair disease
- 汗腺疾病
|
---|
| (M, 710–739) | Musculoskeletal disorders: Myopathy
- Arthropathy
Osteochondropathy
|
---|
| (N, 580–629) | Urologic disease
- 腎病變
- Urinary bladder disease
- Male genital disease
- Breast disease
- Female genital disease
|
---|
| (O, 630–679) | - Complications of pregnancy
- Obstetric labor complication
- Puerperal disorder
|
---|
| (P, 760–779) | |
---|
| (Q, 740–759) | |
---|
| (R, 780–799) | |
---|
| (S/T, 800–999) | - 骨折
- 脫臼
- Sprain
- Strain
- Subluxation
- 頭部外傷
- 胸部创伤
- 毒物
|
---|
|
13X7cF5Z1Hv0,krU VR x6jEYP6tJ E,bG 3ES6ZzwBM
Popular posts from this blog
Ramiro Burr's New Blog - to go back: www.ramiroburr.com From Latin rock to reggaeton, boleros to blues,Tex-Mex to Tejano, conjunto to corridos and beyond, Ramiro Burr has it covered. If you have a new CD release, a trivia question or are looking for tour info, post a message here or e-mail Ramiro directly at: musicreporter@gmail.com Top Tejano songwriter Luis Silva dead of heart attack at 64 By Ramiro Burr on October 23, 2008 8:40 AM | Permalink | Comments (12) | TrackBacks (0) UPDATE: Luis Silva Funeral Service details released Visitation 4-9 p.m. Saturday, Rosary service 6 p.m. Saturday at Porter Loring, 1101 McCullough Ave Funeral Service 10:30 a.m. Monday St. Anthony De Padua Catholic Church, Burial Service at Chapel Hills, 7735 Gibbs Sprawl Road. Porter Loring (210) 227-8221 Related New Flash: Irma Laura Lopez: long time record promoter killed in accident NewsFlash: 9:02 a.m. (New comments below) Luis Silva , one of the most well-known ...
1 I having trouble getting my ResourceDictionary.MergedDictionaries to load from app.xaml. My WPF app has a static class with a Main defined and startup object set to it. Within Main I created an instance of App and run it. The override OnStartup fires and the mainwindow.cs InitializeComponent gives the error "Message "Cannot find resource named 'MaterialDesignFloatingActionMiniAccentButton'. If I put the resources in the mainwindow.xaml everything is fine, but I wanted them to load at the app level so I they are not in each page. Any help appreciated. public partial class App protected override void OnStartup(StartupEventArgs e) base.OnStartup(e); var app = new MainWindow(); var context = new MainWindowViewModel(); app.DataContext = context; app.Show(); from the Main.. var app = new App(); app.Run(); app.xaml.. <Application x:Class="GS.Server.App" xmlns="http://schemas.microsoft.com/winfx/2006/xaml/presentation" xmlns:...
up vote 2 down vote favorite There is a clear pattern that show for two separate subsets (set of columns); If one value is missing in a column, values of other columns in the same subset are missing for any row. Here is a visualization of missing data My tries up until now, I used ycimpute library to learn from other values, and applied Iterforest. I noted, score of Logistic regression is so weak (0.6) and thought Iterforest might not learn enough or anyway, except from outer subset which might not be enough? for example the subset with 11 columns might learn from the other columns but not from within it's members, and the same goes for the subset with four columns. This bar plot show better quantity of missings So of course, dealing with missings is better than dropping rows because It would affect my prediction which does contain the same missings quantity relatively. Any better way to deal with these ? [EDIT] The nullity pattern is confirmed: machine-learning cor...