共有原始形質
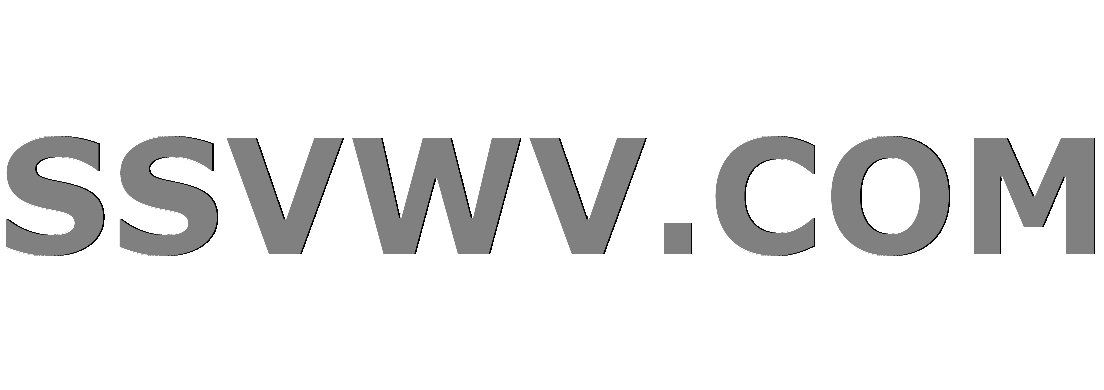
Multi tool use

祖先形質および派生形質の様々なパタンを記述する用語と分岐図 [1]
系統学において、原始形質(げんしけいしつ)あるいは祖先形質(そせんけいしつ)(plesiomorphy)、共有原始形質(きょうゆうげんしけいしつ)あるいは共有祖先形質(きょうゆうそせんけいしつ)(symplesiomorphy or symplesiomorphic character)とは、2つ以上の分類群で共有される祖先的な形質の状態である。原始形質は、通常は派生形質に対して、祖先的な形質状態と言及される。共有原始形質は最も近い共通祖先をもつ、考慮に入れる他の分類群にも共有されうる。したがって、共有原始形質を共有する分類群が、ほかの分類群よりも近縁であることにはならない[2]。共有原始形質(symplesiomorphy)は1950年にドイツ人昆虫学者ヴィリー・ヘニッヒによって造られた語である。
議論
原始形質の概念は派生的な形質状態と祖先的な形質状態を区別せずに、単に形態学的あるいは遺伝学的類似に基づいて、種をグルーピングする危険性を示している。共通祖先から受け継がれた祖先形質は系統樹のいかなる部分にも存在しうるもので、その存在をもって近縁関係を表すことはできない[3]。
有名な例が硬骨魚類と軟骨魚類の鰓呼吸である。硬骨魚類は軟骨魚類よりも肺や皮膚で呼吸をする四足動物に近縁であるが、鰓による呼吸は「魚類」によって共有されており、脊椎動物の共通祖先で存在した鰓呼吸が、四足動物では失ったものと考えられる。しかし共有する形質状態のみに基づけば、硬骨魚類は四足動物よりも軟骨魚類に近縁であるという結論を出してしまうことになる[4]。
関連項目
- 共有派生形質
- 固有派生形質
脚注
^ Roderick D.M. Page; Edward C. Holmes (14 July 2009). Molecular Evolution: A Phylogenetic Approach. John Wiley & Sons. ISBN 978-1-4443-1336-9. https://books.google.com/books?id=p2lWhjuK8m8C.
^ Futuyma, Douglas J. (1998), Evolutionary Biology (3rd ed.), Sunderland, Massachusetts: Sinauer Associates, Inc., p. 95, ISBN 0-87893-189-9
^ Patterson, Colin (1982), “Morphological characters and homology”, in Joysey, Kenneth A; Friday, A. E., Problems in Phylogenetic Reconstruction, Systematics Association Special Volume 21, London: Academic Press, ISBN 0-12-391250-4 .
^ Cracraft, Joel; Donoghue, Michael J. (2004), Assembling the Tree of Life, USA: Oxford University Press, p. 367, ISBN 0-19-517234-5
eUdfteTPqJJe