VAE in Keras: how to define the end-to-end model?
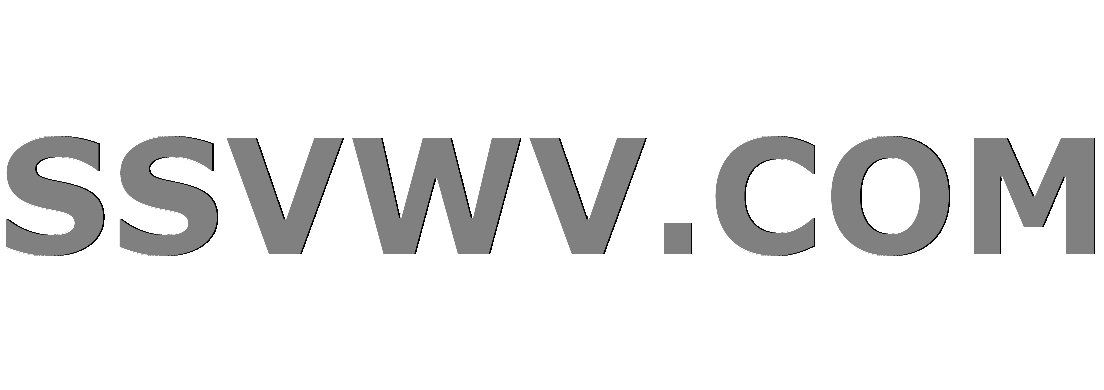
Multi tool use
up vote
1
down vote
favorite
I am learning the tutorial here. My Model part is:
input_img = keras.Input(shape=img_shape)
x = layers.Conv2D(32, (3, 3),
padding='same', activation='relu')(input_img)
...
x = layers.Conv2D(64, (3, 3),
padding='same', activation='relu')(x)
shape_before_flattening = K.int_shape(x)
x = layers.Flatten()(x)
x = layers.Dense(32, activation='relu')(x)
z_mean = layers.Dense(latent_dim)(x)
z_log_var = layers.Dense(latent_dim)(x)
def sampling(args):
...
z = layers.Lambda(sampling)([z_mean, z_log_var])
decoder_input = layers.Input(K.int_shape(z)[1:])
x = layers.Dense(np.prod(shape_before_flattening[1:]),
activation='relu')(decoder_input)
x = layers.Reshape(shape_before_flattening[1:])(x)
x = layers.Conv2DTranspose(32, 3,
padding='same', activation='relu',
strides=(2, 2))(x)
x = layers.Conv2D(1, 3,
padding='same', activation='sigmoid')(x)
# This is our decoder model from letent space to reconstructed images
decoder = Model(decoder_input, x)
# We then apply it to `z` to recover the decoded `z`.
z_decoded = decoder(z)
def vae_loss(self, x, z_decoded):
...
# Fit the end-to-end model
vae = Model(input_img, z_decoded) # vae = Model(input_img, x)
vae.compile(optimizer='rmsprop', loss=vae_loss)
vae.summary()
My question is: the end-to-end is vae = Model(input_img, z_decoded)
or vae = Model(input_img, x)
. Should we compute loss on input_img
and z_decoded
OR between input_img
and x
? Thanks
python keras autoencoder
add a comment |
up vote
1
down vote
favorite
I am learning the tutorial here. My Model part is:
input_img = keras.Input(shape=img_shape)
x = layers.Conv2D(32, (3, 3),
padding='same', activation='relu')(input_img)
...
x = layers.Conv2D(64, (3, 3),
padding='same', activation='relu')(x)
shape_before_flattening = K.int_shape(x)
x = layers.Flatten()(x)
x = layers.Dense(32, activation='relu')(x)
z_mean = layers.Dense(latent_dim)(x)
z_log_var = layers.Dense(latent_dim)(x)
def sampling(args):
...
z = layers.Lambda(sampling)([z_mean, z_log_var])
decoder_input = layers.Input(K.int_shape(z)[1:])
x = layers.Dense(np.prod(shape_before_flattening[1:]),
activation='relu')(decoder_input)
x = layers.Reshape(shape_before_flattening[1:])(x)
x = layers.Conv2DTranspose(32, 3,
padding='same', activation='relu',
strides=(2, 2))(x)
x = layers.Conv2D(1, 3,
padding='same', activation='sigmoid')(x)
# This is our decoder model from letent space to reconstructed images
decoder = Model(decoder_input, x)
# We then apply it to `z` to recover the decoded `z`.
z_decoded = decoder(z)
def vae_loss(self, x, z_decoded):
...
# Fit the end-to-end model
vae = Model(input_img, z_decoded) # vae = Model(input_img, x)
vae.compile(optimizer='rmsprop', loss=vae_loss)
vae.summary()
My question is: the end-to-end is vae = Model(input_img, z_decoded)
or vae = Model(input_img, x)
. Should we compute loss on input_img
and z_decoded
OR between input_img
and x
? Thanks
python keras autoencoder
add a comment |
up vote
1
down vote
favorite
up vote
1
down vote
favorite
I am learning the tutorial here. My Model part is:
input_img = keras.Input(shape=img_shape)
x = layers.Conv2D(32, (3, 3),
padding='same', activation='relu')(input_img)
...
x = layers.Conv2D(64, (3, 3),
padding='same', activation='relu')(x)
shape_before_flattening = K.int_shape(x)
x = layers.Flatten()(x)
x = layers.Dense(32, activation='relu')(x)
z_mean = layers.Dense(latent_dim)(x)
z_log_var = layers.Dense(latent_dim)(x)
def sampling(args):
...
z = layers.Lambda(sampling)([z_mean, z_log_var])
decoder_input = layers.Input(K.int_shape(z)[1:])
x = layers.Dense(np.prod(shape_before_flattening[1:]),
activation='relu')(decoder_input)
x = layers.Reshape(shape_before_flattening[1:])(x)
x = layers.Conv2DTranspose(32, 3,
padding='same', activation='relu',
strides=(2, 2))(x)
x = layers.Conv2D(1, 3,
padding='same', activation='sigmoid')(x)
# This is our decoder model from letent space to reconstructed images
decoder = Model(decoder_input, x)
# We then apply it to `z` to recover the decoded `z`.
z_decoded = decoder(z)
def vae_loss(self, x, z_decoded):
...
# Fit the end-to-end model
vae = Model(input_img, z_decoded) # vae = Model(input_img, x)
vae.compile(optimizer='rmsprop', loss=vae_loss)
vae.summary()
My question is: the end-to-end is vae = Model(input_img, z_decoded)
or vae = Model(input_img, x)
. Should we compute loss on input_img
and z_decoded
OR between input_img
and x
? Thanks
python keras autoencoder
I am learning the tutorial here. My Model part is:
input_img = keras.Input(shape=img_shape)
x = layers.Conv2D(32, (3, 3),
padding='same', activation='relu')(input_img)
...
x = layers.Conv2D(64, (3, 3),
padding='same', activation='relu')(x)
shape_before_flattening = K.int_shape(x)
x = layers.Flatten()(x)
x = layers.Dense(32, activation='relu')(x)
z_mean = layers.Dense(latent_dim)(x)
z_log_var = layers.Dense(latent_dim)(x)
def sampling(args):
...
z = layers.Lambda(sampling)([z_mean, z_log_var])
decoder_input = layers.Input(K.int_shape(z)[1:])
x = layers.Dense(np.prod(shape_before_flattening[1:]),
activation='relu')(decoder_input)
x = layers.Reshape(shape_before_flattening[1:])(x)
x = layers.Conv2DTranspose(32, 3,
padding='same', activation='relu',
strides=(2, 2))(x)
x = layers.Conv2D(1, 3,
padding='same', activation='sigmoid')(x)
# This is our decoder model from letent space to reconstructed images
decoder = Model(decoder_input, x)
# We then apply it to `z` to recover the decoded `z`.
z_decoded = decoder(z)
def vae_loss(self, x, z_decoded):
...
# Fit the end-to-end model
vae = Model(input_img, z_decoded) # vae = Model(input_img, x)
vae.compile(optimizer='rmsprop', loss=vae_loss)
vae.summary()
My question is: the end-to-end is vae = Model(input_img, z_decoded)
or vae = Model(input_img, x)
. Should we compute loss on input_img
and z_decoded
OR between input_img
and x
? Thanks
python keras autoencoder
python keras autoencoder
asked Nov 11 at 1:31
BAE
2,54962762
2,54962762
add a comment |
add a comment |
1 Answer
1
active
oldest
votes
up vote
0
down vote
x is changing throughout the model, where x = layers.Conv2D(1, 3,padding='same', activation='sigmoid')(x)
you set x
to be the last layer of your decoder model.
When doing z_decoded = decoder(z)
you chain your decoder straight after the encoder, z_decoded
is actually the output layer of your decoder, thus, the same x
as earlier. Also, you create the link between the actual input and the output.
Computing the loss would yield the same results on both (as they both represent the same layer).
In short - Both vae = Model(input_img, z_decoded)
and vae = Model(input_img, x)
are the end to end model, i would suggest using the z_decoded version, for readability.
add a comment |
1 Answer
1
active
oldest
votes
1 Answer
1
active
oldest
votes
active
oldest
votes
active
oldest
votes
up vote
0
down vote
x is changing throughout the model, where x = layers.Conv2D(1, 3,padding='same', activation='sigmoid')(x)
you set x
to be the last layer of your decoder model.
When doing z_decoded = decoder(z)
you chain your decoder straight after the encoder, z_decoded
is actually the output layer of your decoder, thus, the same x
as earlier. Also, you create the link between the actual input and the output.
Computing the loss would yield the same results on both (as they both represent the same layer).
In short - Both vae = Model(input_img, z_decoded)
and vae = Model(input_img, x)
are the end to end model, i would suggest using the z_decoded version, for readability.
add a comment |
up vote
0
down vote
x is changing throughout the model, where x = layers.Conv2D(1, 3,padding='same', activation='sigmoid')(x)
you set x
to be the last layer of your decoder model.
When doing z_decoded = decoder(z)
you chain your decoder straight after the encoder, z_decoded
is actually the output layer of your decoder, thus, the same x
as earlier. Also, you create the link between the actual input and the output.
Computing the loss would yield the same results on both (as they both represent the same layer).
In short - Both vae = Model(input_img, z_decoded)
and vae = Model(input_img, x)
are the end to end model, i would suggest using the z_decoded version, for readability.
add a comment |
up vote
0
down vote
up vote
0
down vote
x is changing throughout the model, where x = layers.Conv2D(1, 3,padding='same', activation='sigmoid')(x)
you set x
to be the last layer of your decoder model.
When doing z_decoded = decoder(z)
you chain your decoder straight after the encoder, z_decoded
is actually the output layer of your decoder, thus, the same x
as earlier. Also, you create the link between the actual input and the output.
Computing the loss would yield the same results on both (as they both represent the same layer).
In short - Both vae = Model(input_img, z_decoded)
and vae = Model(input_img, x)
are the end to end model, i would suggest using the z_decoded version, for readability.
x is changing throughout the model, where x = layers.Conv2D(1, 3,padding='same', activation='sigmoid')(x)
you set x
to be the last layer of your decoder model.
When doing z_decoded = decoder(z)
you chain your decoder straight after the encoder, z_decoded
is actually the output layer of your decoder, thus, the same x
as earlier. Also, you create the link between the actual input and the output.
Computing the loss would yield the same results on both (as they both represent the same layer).
In short - Both vae = Model(input_img, z_decoded)
and vae = Model(input_img, x)
are the end to end model, i would suggest using the z_decoded version, for readability.
answered Nov 11 at 7:11
Or Dinari
1,014321
1,014321
add a comment |
add a comment |
Sign up or log in
StackExchange.ready(function ()
StackExchange.helpers.onClickDraftSave('#login-link');
);
Sign up using Google
Sign up using Facebook
Sign up using Email and Password
Post as a guest
Required, but never shown
StackExchange.ready(
function ()
StackExchange.openid.initPostLogin('.new-post-login', 'https%3a%2f%2fstackoverflow.com%2fquestions%2f53245079%2fvae-in-keras-how-to-define-the-end-to-end-model%23new-answer', 'question_page');
);
Post as a guest
Required, but never shown
Sign up or log in
StackExchange.ready(function ()
StackExchange.helpers.onClickDraftSave('#login-link');
);
Sign up using Google
Sign up using Facebook
Sign up using Email and Password
Post as a guest
Required, but never shown
Sign up or log in
StackExchange.ready(function ()
StackExchange.helpers.onClickDraftSave('#login-link');
);
Sign up using Google
Sign up using Facebook
Sign up using Email and Password
Post as a guest
Required, but never shown
Sign up or log in
StackExchange.ready(function ()
StackExchange.helpers.onClickDraftSave('#login-link');
);
Sign up using Google
Sign up using Facebook
Sign up using Email and Password
Sign up using Google
Sign up using Facebook
Sign up using Email and Password
Post as a guest
Required, but never shown
Required, but never shown
Required, but never shown
Required, but never shown
Required, but never shown
Required, but never shown
Required, but never shown
Required, but never shown
Required, but never shown
1gjpsmonhAR,nyeDxlb Bg1RpF6zbC5L6AyYiHstFk6mFh3x