オプティマテス
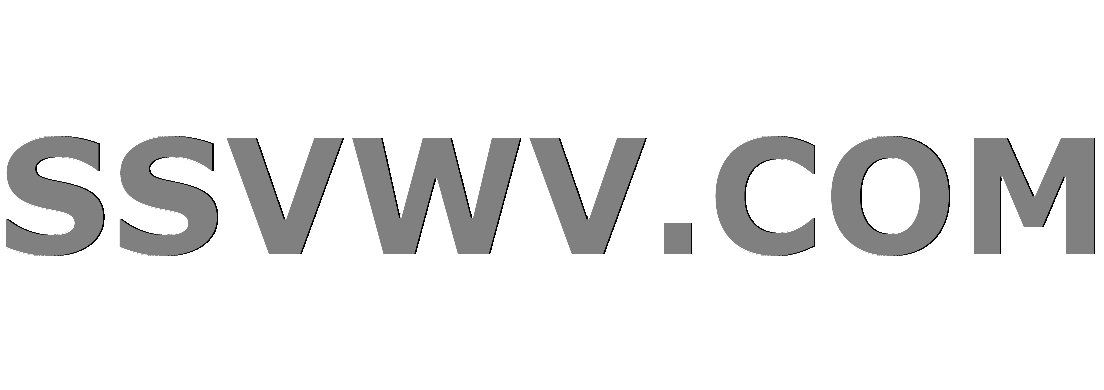
Multi tool useオプティマテス(羅: optimates)は、共和政ローマ末期の政治一派。日本語では閥族派、元老院派と呼ばれる。
概要
共和政ローマでは、長年にわたって元老院主導のもと政治が行われてきた。オプティマテスは元老院主導による政治体制の維持を図った者の総称であり、その名はラテン語の「良い」の最上級・オプティムス(optimus)に由来する。そのため「最良の人士」と訳されることもある。
他にも「良き人々」を意味するボニ(boni)の表記も見られるが、この表記はオプティマテスの記した資料に登場するものであることに留意する必要がある。
オプティマテスと呼ばれる人には、元老院の多数派を占めるノビレス(新貴族)が多く見られるが、ルキウス・コルネリウス・スッラや小カトーと並び代表的なオプティマテスとされるマルクス・トゥッリウス・キケロはノウス・ホモである。
歴史
共和政末期のローマでは、相次ぐ戦争とそれに伴う領地拡大により経済構造が大きく変化し政治に歪みが生じてきていた。具体的に言えば格差の拡大に伴う平民の困窮・没落である。そしてそれに伴う、軍事力の低下である(当時のローマ軍は一定以上の資産を持つ市民からの徴兵制で成立していた)。この事態を受け、グラックス兄弟は平民を救済すべく改革に乗り出した。
この改革に対し、元老院の多数を占めるノビレスは反発する。加えてグラックス兄弟は護民官という立場のもと、法案を全市民による市民集会であるケントゥリア民会・トリブス民会ではなくて、平民のみの市民集会であるプレブス民会で成立させるという手段に出たため、元老院との対立はますます深まり、結果としてグラックス兄弟とその一派は粛清されて改革は失敗に終わる。
しかしグラックス兄弟以降も、平民の支持を基盤として元老院に対抗しようとするポプラレスが相次いで出現した。その中から次第にガイウス・マリウスが台頭してくる。マリウスは自ら行った軍の改革(彼は徴兵制を志願兵制に切り替え、困窮した平民を軍に雇用して救済しつつ軍事力を回復させる政策をとった)で得た強大な軍事力と民衆の支持を背景に政治権力を握ろうとした。元老院はそれを阻止しようとしたが、ローマの軍事力を建て直す必要性から平民の救済は急務であり、結局はマリウスらポプラレスが権力を掌握した。
マリウスの死後、彼の政敵であるスッラは独裁官に就任、このときにオプティマテスの政治的思想は最大限適用された。スッラのもとで民会は政治力のほとんどを奪われ、元老院議員は300人から600人に定員を増やした。そしてポプラレスは多数粛清され、オプティマテスは最盛期を迎える。しかしながらスッラの独裁政治は、元老院主導の寡頭制を理想とする多くのオプティマテスにとっても悪夢であり、スッラの死後に彼の構築した体制は徐々に崩れていく。皮肉にも独裁体制をとらねば、もはや元老院主導を目指す政治派閥による体制は維持出来ず、これはオプティマテスの政治的限界を意味していた。
その後、マリウスの義理の甥にあたるガイウス・ユリウス・カエサルの台頭とともにポプラレスは復活を遂げるが、オプティマテスも依然として勢力を保っていた。カエサルはローマ内戦の末、オプティマテスを打ち破り権力を掌握し、かつて頓挫したグラックス兄弟とほとんど同じ改革を実行した。だが、後にカエサルの許しを得て元老院議員の身分に留まる者もいた(後にカエサルを暗殺するマルクス・ユニウス・ブルトゥスもその1人である)。
カエサル暗殺後、第二回三頭政治が始まり、そこでオプティマテスは多数粛清され政治的な終焉を迎えた。以降、ローマの政治はカエサルの後継を巡るポプラレス同士の権力闘争へと移行する。
rSHe0UU73xYuHtHUgA61fR76yXIe7Yvmy Mms55zKJvdaM2s4bPUUlz
Popular posts from this blog
Ramiro Burr's New Blog - to go back: www.ramiroburr.com From Latin rock to reggaeton, boleros to blues,Tex-Mex to Tejano, conjunto to corridos and beyond, Ramiro Burr has it covered. If you have a new CD release, a trivia question or are looking for tour info, post a message here or e-mail Ramiro directly at: musicreporter@gmail.com Top Tejano songwriter Luis Silva dead of heart attack at 64 By Ramiro Burr on October 23, 2008 8:40 AM | Permalink | Comments (12) | TrackBacks (0) UPDATE: Luis Silva Funeral Service details released Visitation 4-9 p.m. Saturday, Rosary service 6 p.m. Saturday at Porter Loring, 1101 McCullough Ave Funeral Service 10:30 a.m. Monday St. Anthony De Padua Catholic Church, Burial Service at Chapel Hills, 7735 Gibbs Sprawl Road. Porter Loring (210) 227-8221 Related New Flash: Irma Laura Lopez: long time record promoter killed in accident NewsFlash: 9:02 a.m. (New comments below) Luis Silva , one of the most well-known ...
1 I having trouble getting my ResourceDictionary.MergedDictionaries to load from app.xaml. My WPF app has a static class with a Main defined and startup object set to it. Within Main I created an instance of App and run it. The override OnStartup fires and the mainwindow.cs InitializeComponent gives the error "Message "Cannot find resource named 'MaterialDesignFloatingActionMiniAccentButton'. If I put the resources in the mainwindow.xaml everything is fine, but I wanted them to load at the app level so I they are not in each page. Any help appreciated. public partial class App protected override void OnStartup(StartupEventArgs e) base.OnStartup(e); var app = new MainWindow(); var context = new MainWindowViewModel(); app.DataContext = context; app.Show(); from the Main.. var app = new App(); app.Run(); app.xaml.. <Application x:Class="GS.Server.App" xmlns="http://schemas.microsoft.com/winfx/2006/xaml/presentation" xmlns:...
up vote 2 down vote favorite There is a clear pattern that show for two separate subsets (set of columns); If one value is missing in a column, values of other columns in the same subset are missing for any row. Here is a visualization of missing data My tries up until now, I used ycimpute library to learn from other values, and applied Iterforest. I noted, score of Logistic regression is so weak (0.6) and thought Iterforest might not learn enough or anyway, except from outer subset which might not be enough? for example the subset with 11 columns might learn from the other columns but not from within it's members, and the same goes for the subset with four columns. This bar plot show better quantity of missings So of course, dealing with missings is better than dropping rows because It would affect my prediction which does contain the same missings quantity relatively. Any better way to deal with these ? [EDIT] The nullity pattern is confirmed: machine-learning cor...