类Unix系统
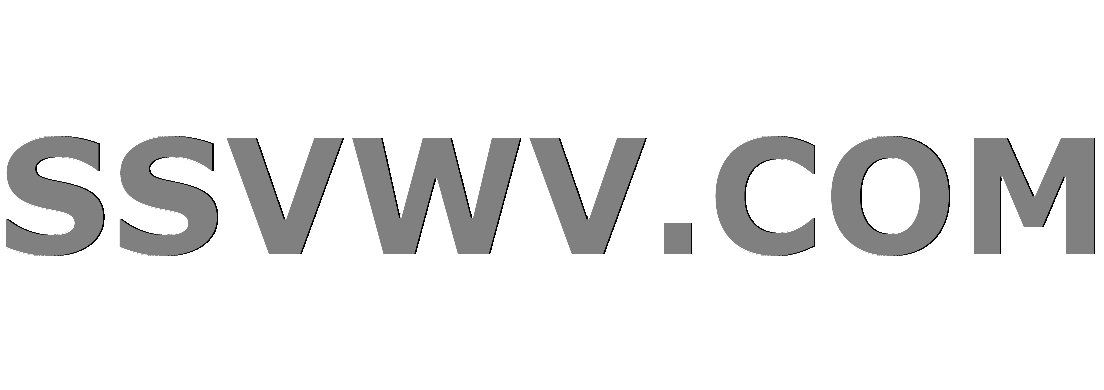
Multi tool use

数种「类UNIX操作系统」的相互关系图
类Unix系统(英语:Unix-like)经常被称为 UN*X 或 *nix,指各种Unix的衍生系统,比如FreeBSD、OpenBSD、SUN公司的Solaris,以及各种与传统Unix类似的系统,例如Minix、Linux、QNX等。它们虽然有的是自由软件,有的是私有软件,但都相当程度地继承了原始UNIX的特性,有许多相似处,并且都在一定程度上遵守POSIX规范。
UNIX的商標權由國際開放標準組織所擁有,只有符合單一UNIX規範的UNIX系統才能使用UNIX這個名稱,否則只能稱為類UNIX(UNIX-like)。
目录
1 分類和例子
1.1 自由软件/開源軟件
1.2 私有软件
2 参考文献
3 參見
分類和例子
自由软件/開源軟件
Agnix(教育用)
386BSD及其子類(BSD系统):
FreeBSD及其子類:- ClosedBSD
- Apple Darwin
- DragonFly BSD
- GNU/kFreeBSD
- PC-BSD
NetBSD及其子類:- GNU/kNetBSD
OpenBSD及其子類:- ekkoBSD
- MicroBSD
- MirOS BSD
GNU- GNU Hurd
- GNU/kFreeBSD
- GNU/kNetBSD
Linux(又称GNU/Linux)- GNU/OpenSolaris
- LUnix
MINIX及其子類:- Minix-vmd
- MINIX 3
OpenSolaris - 建基於System V- Phoenix-RTOS
九号计划:Unix的后继者,采用UNIX设计与哲学,但更一致地套用至整个分布式系统,功能上并不完全相同。
Inferno:Plan 9衍生出的分布式操作系统,原本由贝尔实验室开发,现在被Vita Nuova拥有。
Plan B:Plan 9衍生出的分布式操作系统[1])
Syllable:99% POSIX依從
VSTa:大致POSIX依從
Maemo:诺基亚的开源系统
私有软件
IBM AIX* - 建基於System V Release 3
HP HP-UX*
SGI IRIX*
Apple macOS - 建基於Apple Darwin (自 10.5 開始符合單一UNIX規範)
Apple iOS - 建基於Apple Darwin- LynxOS RTOS
QNX - 全部重写,没有UNIX相关的代码
SkyOS - 大致POSIX依從
Sun
SunOS - 建基於BSD
Solaris* - 建基於System V Release 4
Compaq Tru64* - 建基於OSF/1
Microsoft Xenix- VxWorks
- * UNIX® branded systems
参考文献
參見
- 操作系统列表
|
Nm1c,P,yfFTm3 5Ue b8l6JK,Hu6AoprxK Q7Gf8d