娃娃
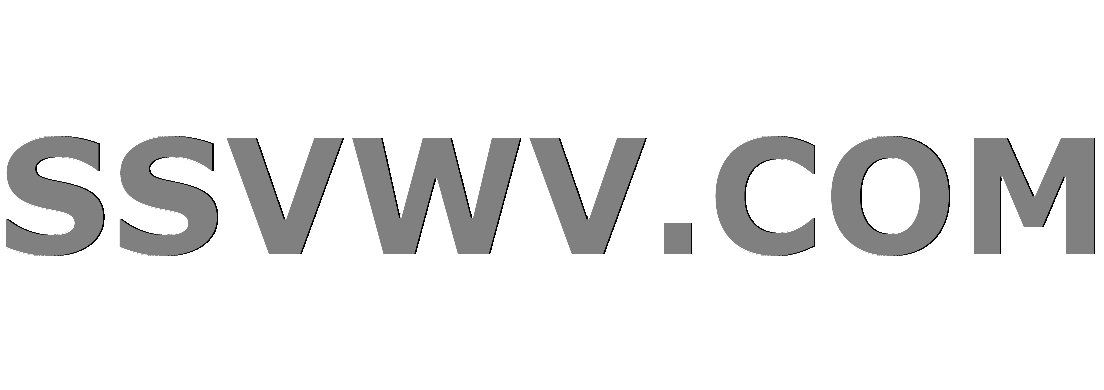
Multi tool use
本文介紹的是可用作玩具的布偶。關於別名「娃娃」的人物,請見「
娃娃 (消歧义)」。
 | 查询維基詞典中的doll。 |
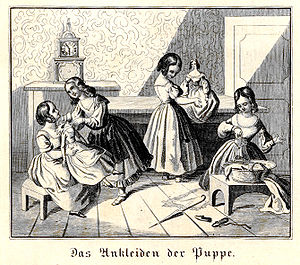
Das Ankleiden der Puppe (英語: Dressing the Doll),1844年
娃娃、玩偶一詞泛指模仿人或動物造型的賞玩物品,其中形若人類的稱為人偶。人偶、玩偶的外型面貌,從抽象到擬真,涵蓋不同種族、年齡、性別的外型,因各國、各民族的需求與文化背景而發展出地域特性及文化特色。製作材料包括玻璃、金屬、土、木、布、陶瓷、樹脂等。
在漢語中,娃娃也有小孩的意思,故亦以此指稱按比例縮小的人偶。
用途
祭典儀式
在古老的社會裡,人偶往往是真人在祭典儀式中的替身。後來這種人偶做得益發精美,而進一步發展成裝飾品及玩具。
賞玩遊樂
泛稱用來作給兒童的玩具,用以安慰小孩、供其玩耍。有些娃娃的設計會反映社會的流行與思潮,並期望培養小孩對社會角色的認同感。此外也有大人進行收藏。
研究教學
有些擬真娃娃會全面或部份地講求跟人類一樣的精細,用以教學、研究或特定用途。
種類
- 洋娃娃(Fashion doll,直釋:時尚娃娃),與可動人形最大的分別是只是身軀或頭部是塑膠,衣物和太多數頭髮就用布料和人造纖維製作。最著名品牌有:
- 芭比娃娃
- 貝姿娃娃(Bratz)
- 精靈高中(Monster High)
- 迪士尼公主
- 莉卡娃娃(Licca)
- 珍妮娃娃
- 芙拉娃娃
- MIMI娃娃(The MIMI doll)
- 佩莉普娃娃(Pullip)
- 仙蒂娃娃(Sindy)
- 魔法俏佳人
- 可兒娃娃(Kurhn)
- 布萊絲
- 填充娃娃(毛絨玩具、布娃娃)
- 李岷洋•羅斯福•泰迪(dog doll)
- 娃娃屋人偶(Toy House Doll)
- 波莉口袋(Polly Pocket)
- 森林家族(Forest Homestea)
隆德比(Lundby)
- 摩比
- 萬能麥斯
- 女生派對驚喜(Miss Party Surprise)
- 溫蒂娃娃(Wendy)
- 皮威娃娃(Pee Wee)
- 紙娃娃(Paper doll)
- 非洲娃娃(African doll)
晴天娃娃(日語:てるてる坊主,意指晴天和尚)
巫毒娃娃(Voodoo doll)
- 霍皮娃娃(Hopi Kachina dolls)
- Q比娃娃(Kewpie)
- 美國女孩(American Girls)
- 樂樂天使(Lalaloopsy)
- Ddung娃娃(Ddung)
- 胡桃鉗人偶(Nutcracker)
- 小錫兵(Tin soldiers)
- 不倒翁
- 日本娃娃
- 俄羅斯套娃
- 大頭娃娃、搖頭娃娃(Bobblehead doll)
- 名人娃娃(Celebrity doll)
- 企業娃娃「公仔」(Enterprise doll)
- 大同寶寶
- 佐藤象(Sato Elephant)
- 王子麵寶寶(Prince Instant Noodles)
- 新力寶寶(Sony)
- 可動人像(Action Figure),這名詞是為1964年孩之寶推出的特種部隊G.I. Joe所創的新詞,目的是令男孩玩家顯得少些娘娘腔。現在亞洲的可動人形通常都是以ACG美少女為主,而歐美的主題就以電影、超級英雄等作題材。
- 義勇羣英
- 變形金剛
- 小馬寶莉
- 超人系列
- 假面騎士系列
球體關節人形
- SD娃娃
- figma
- S.H.Figuarts
- 黏土人
- 包膠素體
手辦(人形模型或、手辦模型)
人形(figure,起初指的是人形的雕像和塑像,但至今意义逐渐转变至指代所有特定人物的人物模型)
- 手辦
参考文献
规范控制 | - GND: 4047870-1
- NDL: 00568514
|
---|
|
PTamf3,T2VDFwB2UuydCFWCPNP6UwcTlfnQTQU,pJUI6T62,OlGql6c9T0S,DR5mCZYAy,CcP1u,kd0gyioeGQuLLoEMpZEo
Popular posts from this blog
Ramiro Burr's New Blog - to go back: www.ramiroburr.com From Latin rock to reggaeton, boleros to blues,Tex-Mex to Tejano, conjunto to corridos and beyond, Ramiro Burr has it covered. If you have a new CD release, a trivia question or are looking for tour info, post a message here or e-mail Ramiro directly at: musicreporter@gmail.com Top Tejano songwriter Luis Silva dead of heart attack at 64 By Ramiro Burr on October 23, 2008 8:40 AM | Permalink | Comments (12) | TrackBacks (0) UPDATE: Luis Silva Funeral Service details released Visitation 4-9 p.m. Saturday, Rosary service 6 p.m. Saturday at Porter Loring, 1101 McCullough Ave Funeral Service 10:30 a.m. Monday St. Anthony De Padua Catholic Church, Burial Service at Chapel Hills, 7735 Gibbs Sprawl Road. Porter Loring (210) 227-8221 Related New Flash: Irma Laura Lopez: long time record promoter killed in accident NewsFlash: 9:02 a.m. (New comments below) Luis Silva , one of the most well-known ...
政治主題的一部分 政党政治 政治光譜 左派 極左 中間偏左 中间派 中間偏左 激進中間 ( 英语 : Radical centrism ) 中間偏右 右派 中間偏右 極右 政綱 極端 激进 ( 英语 : Political radicalism ) 温和 ( 英语 : Moderate ) 改良 混合 ( 英语 : Syncretic politics ) 第三位置 保守 原教旨 反动 政党制度 无党制 ( 英语 : Non-partisan democracy ) 一党制 一党优...
Skip Main Navigation Links Jump to Footer var prs = rt: function(label,ts) ts==0?ts:new Date().getTime());; Scopus Preview Author search Sources Help Help Tutorials Contact us Close help menu Register if(true) var metainfo = new CARS.metadata(); metainfo.elementId = 'login_loginlink_expand'; metainfo.action = 'toggle'; metainfo.dependency = 'login_loginlink_expand,login_loginlink_collapse,login_pop_container'; metainfo.name = 'login_loginlink_expand'; CARS_dataList.push(metainfo); if(true) var metainfo = new CARS.metadata(); metainfo.elementId = 'login_loginlink_collapse'; metainfo.action = 'toggle'; metainfo.dependency = 'login_loginlink_collapse,login_loginlink_expand,login_pop_container'; metainfo.name = 'login_loginlink_collapse'; CARS_dataList.push(metainfo); Login if(true) var metainfo = new CARS....