オランダ総督
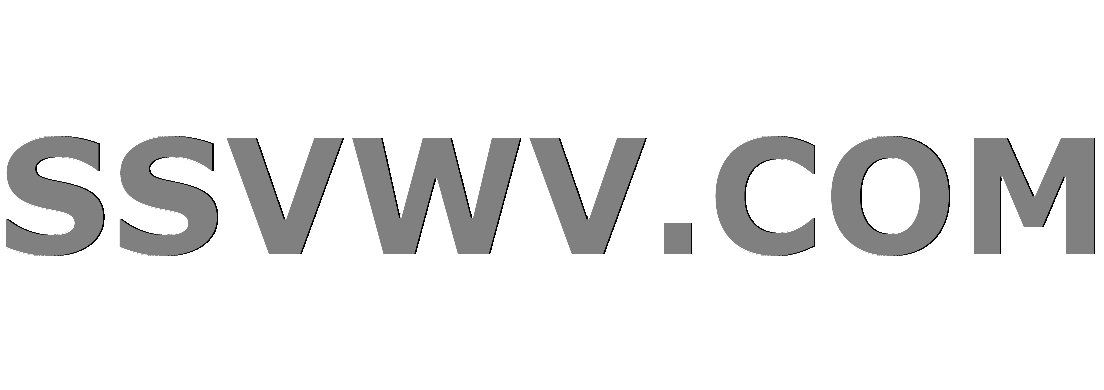
Multi tool use | この記事は検証可能な参考文献や出典が全く示されていないか、不十分です。 出典を追加して記事の信頼性向上にご協力ください。(2016年3月)
|
オランダ総督(オランダそうとく、蘭: stadhouder, 英: stadtholder)は、広義には16世紀から18世紀にかけてのネーデルラント連邦共和国(オランダ王国の前身)における各州の首長。狭義にはその中でも特に有力で、ゼーラント州など他の州の総督も兼ね、事実上の世襲君主として君臨したホラント州の総督を指す。
ただしこのstadtholderの訳語に、植民地の長官を意味するgovernorの訳語としてすでに定着している「総督」を充てるのは、意味上の混乱を招きやすく不都合なため、今日ではオランダ総督に代えてオランダ統領と表現することも見られるようになった。
概要
元は15世紀以降、ネーデルラント17州を統治していたハプスブルク家の君主が、有力なネーデルラント貴族を(本来の意味での)総督(あるいは知事)に任命し、各州の統治を委ねたことが起源である。ネーデルラント随一の貴族であり、後に八十年戦争を主導したオラニエ公ウィレム1世も、はじめはスペイン王フェリペ2世によって総督に任じられていた。
1568年に始まる八十年戦争でネーデルラント各州はフェリペ2世に対し反乱を起こし、やがて北部7州は新たな君主を戴くことなく連邦共和国として独立を果たした。連邦共和国の体制においては、7つの州の議会それぞれが君主に代わって自ら総督を指名した。総督は州の首長として議会を主導し、法の執行を監督し、役職者の任免を行なった。総督の地位は初期を除けばほとんどオラニエ=ナッサウ家の当主であるオラニエ公、およびその傍系ナッサウ=ディーツ家の世襲であった。ウィレム1世以来歴代のオラニエ公のほとんどは、連邦の7州の中心的存在であるホラント州の他4 - 5の州の総督を兼ね、残りの州の総督はウィレム1世の弟ナッサウ=ディレンブルク伯ヨハン6世の家系であるナッサウ=ディーツ家が占めた。ホラント州とゼーラント州の総督(ウィレム1世以後常に兼任された)は慣習的に連邦議会から陸海軍総司令官にも任命されており、その権力は大きく、実質的に君主に近い存在であった。
こうした総督=オラニエ公の権勢に対して反発する勢力もあり、イングランド王を兼ねたウィレム3世の総督時代(1672年 - 1702年)を挟んで1650年から1747年の間に、2度にわたって主要な州で総督を置かなかった時代がある(無総督時代)。しかし1747年、オーストリア継承戦争に巻き込まれてフランス軍の侵攻を受けた連邦共和国は、イギリスの後押しもあって、ウィレム4世を7州全ての総督に指名し、その地位の世襲を宣言した。この全州総督の地位はウィレム5世に継承され、フランス革命戦争の中で連邦共和国が崩壊する1795年まで続いた。
脚注
![[icon]](//upload.wikimedia.org/wikipedia/commons/thumb/1/1c/Wiki_letter_w_cropped.svg/20px-Wiki_letter_w_cropped.svg.png) | この節の加筆が望まれています。 主に: 脚注形式での出典の明記 (2016年3月)
|
参考文献
![[icon]](//upload.wikimedia.org/wikipedia/commons/thumb/1/1c/Wiki_letter_w_cropped.svg/20px-Wiki_letter_w_cropped.svg.png) | この節の加筆が望まれています。 (2016年3月)
|
関連項目
E05N108BcO,UTF0tDu6 4nQhkezChfZ1URf5p,aRGiBlJyTUKKzGbB8 wC870yEb2Ul 1YOpZIzeW4s9Wql1E7Vxfw
Popular posts from this blog
Ramiro Burr's New Blog - to go back: www.ramiroburr.com From Latin rock to reggaeton, boleros to blues,Tex-Mex to Tejano, conjunto to corridos and beyond, Ramiro Burr has it covered. If you have a new CD release, a trivia question or are looking for tour info, post a message here or e-mail Ramiro directly at: musicreporter@gmail.com Top Tejano songwriter Luis Silva dead of heart attack at 64 By Ramiro Burr on October 23, 2008 8:40 AM | Permalink | Comments (12) | TrackBacks (0) UPDATE: Luis Silva Funeral Service details released Visitation 4-9 p.m. Saturday, Rosary service 6 p.m. Saturday at Porter Loring, 1101 McCullough Ave Funeral Service 10:30 a.m. Monday St. Anthony De Padua Catholic Church, Burial Service at Chapel Hills, 7735 Gibbs Sprawl Road. Porter Loring (210) 227-8221 Related New Flash: Irma Laura Lopez: long time record promoter killed in accident NewsFlash: 9:02 a.m. (New comments below) Luis Silva , one of the most well-known ...
1 I having trouble getting my ResourceDictionary.MergedDictionaries to load from app.xaml. My WPF app has a static class with a Main defined and startup object set to it. Within Main I created an instance of App and run it. The override OnStartup fires and the mainwindow.cs InitializeComponent gives the error "Message "Cannot find resource named 'MaterialDesignFloatingActionMiniAccentButton'. If I put the resources in the mainwindow.xaml everything is fine, but I wanted them to load at the app level so I they are not in each page. Any help appreciated. public partial class App protected override void OnStartup(StartupEventArgs e) base.OnStartup(e); var app = new MainWindow(); var context = new MainWindowViewModel(); app.DataContext = context; app.Show(); from the Main.. var app = new App(); app.Run(); app.xaml.. <Application x:Class="GS.Server.App" xmlns="http://schemas.microsoft.com/winfx/2006/xaml/presentation" xmlns:...
up vote 2 down vote favorite There is a clear pattern that show for two separate subsets (set of columns); If one value is missing in a column, values of other columns in the same subset are missing for any row. Here is a visualization of missing data My tries up until now, I used ycimpute library to learn from other values, and applied Iterforest. I noted, score of Logistic regression is so weak (0.6) and thought Iterforest might not learn enough or anyway, except from outer subset which might not be enough? for example the subset with 11 columns might learn from the other columns but not from within it's members, and the same goes for the subset with four columns. This bar plot show better quantity of missings So of course, dealing with missings is better than dropping rows because It would affect my prediction which does contain the same missings quantity relatively. Any better way to deal with these ? [EDIT] The nullity pattern is confirmed: machine-learning cor...